[dropcap style=”fontsize:100px;color:#992211;”]X[/dropcap]ray vision has long seemed like a far-fetched sci-fi fantasy, but over the last decade a team from MIT’s Computer Science and Artificial Intelligence Laboratory (CSAIL) has continually gotten us closer to seeing through walls. Their latest project, ‘RF-Pose’, uses artificial intelligence (AI) to teach wireless devices to sense people’s postures and movement, even from the other side of a wall.
The researchers use a neural network to analyse radio signals that bounce off people’s bodies, and can then create a dynamic stick figure that walks, stops, sits and moves its limbs as the person performs those actions.
The team is currently working with doctors to explore multiple applications in healthcare. “We’ve seen that monitoring patients’ walking speed and ability to do basic activities on their own gives healthcare providers a window into their lives that they didn’t have before, which could be meaningful for a whole range of diseases,” says Professor Dina Katabi, who co-wrote a new paper about the project. “A key advantage of our approach is that patients do not have to wear sensors or remember to charge their devices.”
Besides healthcare, the team says that RF-Pose could also be used for new classes of video game where players move around the house, or even in search-and-rescue missions to help locate survivors.
One challenge the researchers had to address is that most neural networks are trained using data labeled by hand. A neural network trained to identify cats, for example, requires that people look at a big dataset of images and label each one as either ‘cat’ or ‘not cat’. Radio signals, meanwhile, can’t be easily labeled by humans. To address this, they collected examples using both their wireless device and a camera, gathering thousands of images of people performing activities like walking, talking, sitting, opening doors and waiting for elevators.
They then used these images from the camera to extract the stick figures, which they showed to the neural network along with the corresponding radio signal. This combination of examples enabled the system to learn the association between the radio signal and the stick figures of the people in the scene. Post-training, RF-Pose was able to estimate a person’s posture and movements without cameras, using only the wireless reflections that bounce off people’s bodies.
Since cameras can’t see through walls, the network was never explicitly trained on data from the other side of a wall, which is what made it particularly surprising to the MIT team that the network could generalise its knowledge to be able to handle through-wall movement. “If you think of the computer vision system as the teacher, this is a truly fascinating example of the student outperforming the teacher,” says MIT professor Antonio Torralba.
Besides sensing movement, the authors also showed that they could use wireless signals to accurately identify somebody 83 per cent of the time out of a line-up of 100 individuals. This ability could be particularly useful for the application of search-and-rescue operations, when it may be helpful to know the identity of specific people.
For this paper, the model outputs a 2-D stick figure, but the team is also working to create 3-D representations that would be able to reflect even smaller micro-movements. For example, it might be able to see if an older person’s hands are shaking regularly enough that they may want to get a check-up.
“By using this combination of visual data and AI to see through walls, we can enable better scene understanding and smarter environments to live safer, more productive lives,” says lead author Mingmin Zhao.
Source: EurekAlert!
Image: BFI
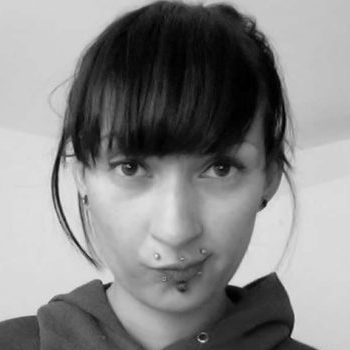
Naila Scargill is the publisher and editor of horror journal Exquisite Terror. Holding a broad editorial background, she has worked with an eclectic variety of content, ranging from film and the counterculture, to political news and finance.